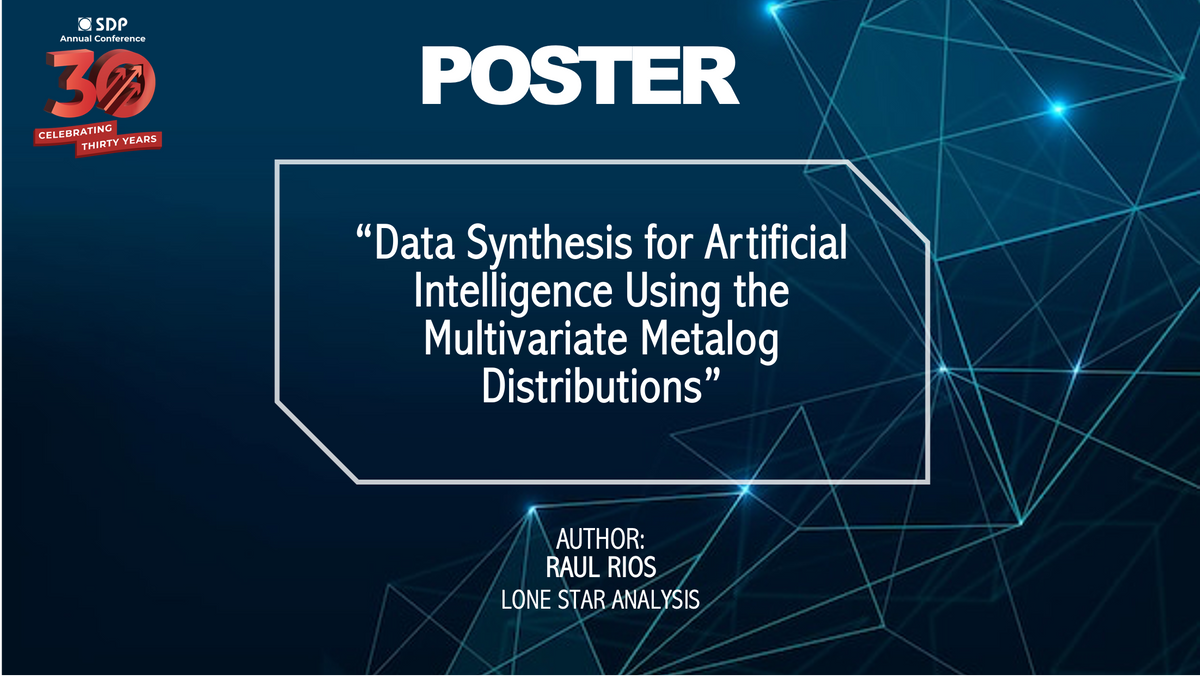
DATA SYNTHESIS FOR ARTIFICIAL INTELLIGENCE USING THE MULTIVARIATE METALOG DISTRIBUTIONS
Author: Raul Rios (Lone Star Analysis)
Abstract:
To achieve high prediction accuracy, common Artificial Intelligence (AI) methods require vast amounts of training datapoints. Such methods, therefore, have diminished usefulness when data is scarce. One option to alleviating data scarcity is to generate new synthetic datapoints that are similar to the original dataset. A potential way to do this is to create a probability distribution fit to the original data and then randomly sample from that distribution to generate synthetic – but realistic – datapoints. A plethora of distributions are available for smooth, continuous data (e.g., normal, Student’s t, Cauchy, logistic, or beta) however, in many cases, it may be unclear which distribution would best capture the reality of the data (shape, skewness, tail behavior, etc.). In addition, the distribution fitting process for these approaches is often complex, subjective, and non-convergent. This paper explores the use of metalog distributions which alleviate these problems by providing an approachable, flexible distribution with closed-form formulations for fitting. We demonstrate the efficacy of metalog distributions in generating synthetic data for training an AI model. We use examples with correlated multi-variate data and measure the impact of statistical copulas for the multivariate metalog. Finally, we compare the metalog results with those from a multi-variate gaussian fit.